<< TK-Brain Model >>
MSA: Maximum Satisfaction Architecture
Introduction
With the advance of the Internet, mankind will become more networked and will have to live together with a number of intelligent autonomous agents. WEB 2.0 is the symbolic term to refer to this trend. The agents would have a variety of purposes, but their ultimate goal would be to ensure a better life for humans. However, useful models for designing such agents have not been provided in the research disciplines, such as Cognitive Sciences and Information Technology. This is mainly because traditional linear and reductionistic approaches would not be appropriate for modeling human beings, or brains, which exhibit a multi-layered structure with nonlinear interconnections (Prigogine, 1997).
Nonlinearity means two important things that affect development of individual brain–society system:
- Dissipative system: A fluctuation of the system caused by an environmental change would trigger creation of a new order or catastrophe, and
- Sensitive Dependence on Initial Condition (SEDIC): A small variation in the initial condition, during one’s infant period, would develop exponentially as one grows up.
MSA: Maximum Satisfaction Architecture
Maximum Satisfaction Architecture (MSA) consists of three parts: 1) happiness goals, i.e., basic living purposes of human beings, 2) human brain, and 3) society, with the aim of providing a basis for designing intelligent autonomous agents that contribute to realizing better living.
- Happiness goals. MSA assumes that the human brain pursues one of the seventeen happiness goals defined by Morris(2006) at every moment, and switches when appropriate by evaluating the current circumstances.
- Society layers. Each of the happiness goals is associated with one or multiple layers of Society: Individual, Family and Community, and Administration and Enterprise. These layers have evolved from the history of human beings. Each layer is associated with its own value reflecting historical development, and thus different sets of happiness goals are relevant.
- Brain layers. The knowledge necessary to achieve the happiness goals is partly acquired and partly inherited. At the level of Conscious layer, knowledge such as formal laws and social mechanisms necessary to deal with administration and enterprise, and formal social norms and common sense to deal with “family and community” and “individual” is acquired. In contrast, knowledge such as basic functions for using language and primitive decision characteristics is inherited. Similarly, at the level of Autonomous-automatic behavior control layer, knowledge such as individual experience and habit is acquired to deal with “family and community” and “individual.” However, as opposed to the inherited knowledge at the Conscious layer, all basic functions that are reproducible by development and bodily experience are inherited in the Autonomous-automatic behavior control layer.
The pieces of knowledge at each layer in the brain are nonlinearly interconnected through individual experience. This implies that individuals that pursue the same goal might have different patterns of activated networks because of SEDIC, and thus the processes to achieve the goal might be different.
An intelligent autonomous agent must be sensitive to the individual differences in the processes to achieve a goal and provide sophisticated support for individuals to achieve that goal.
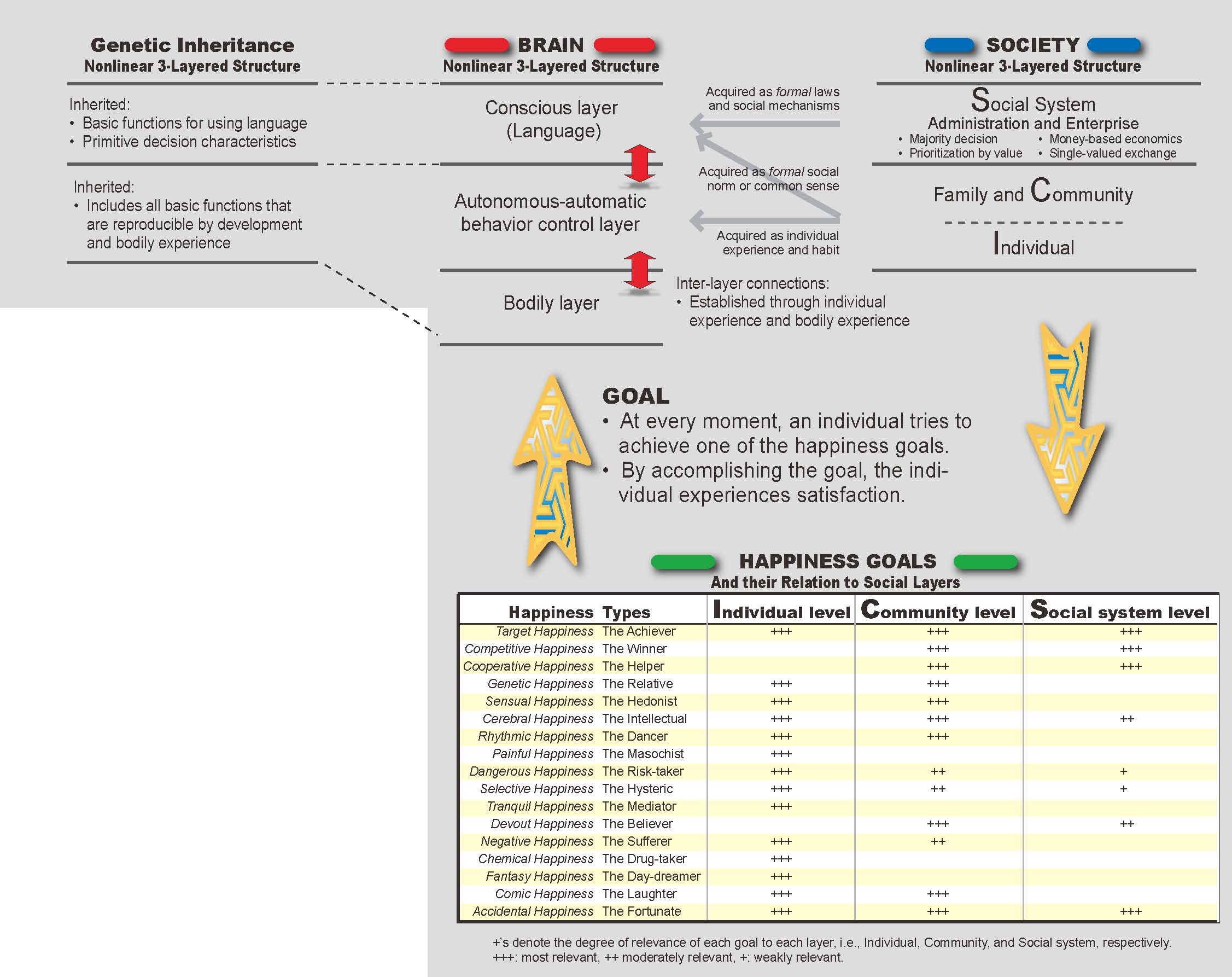
Conditions to make people feel satisfaction
The amount of satisfaction feeling is influenced by the factors that characterize the shape of trajectory of behavioral outcome. There are six critical factors to make people feel satisfaction.
- Change: Perceptual functions work by sensing dynamic changes. Therefore responses while the system is stable are limited. A condition for feeling satisfactory feeling is "change."
- Succession of good results: Successive happiness tends to create memory traces for the best experience and the final outcome of the overall estimation of the events that have lead to successive good results.
- Direction of absolute outcome (denoted as 2 in the Figure): A change to good direction at the end of a series of events tends to create a memory trace of having satisfactory feeling. The degree of the strength of the memory trace would be proportional to the degree of the change towards good direction.
- Amplitude of success (denoted as 1 in the Figure): The greater the difference between the highest event and the lowest event in terms of the degree of the strength of satisfactory feeling, the stronger the strength of memory trace for the entire events including the highest and the lowest. One would create satisfactory feeling as the result of overcoming the lowest evaluated situation and tends to memorize it.
- Absolute amount of outcome (denoted as 3 in the Figure) and Direction of absolute outcome (denoted as 2 in the Figure): When the absolute outcome is acceptable and the contents in working memory at the time of final event are good, they jointly affect the result of estimation of entire events.
- Bad results would not be memorized: When an event occurs that results in bad results, one would strongly react to it when the degree of badness exceeds a certain threshold value. This event would create a memory trace for must-avoid-event. However, memory traces for the events that would be exerted while recovering from the bad situation tend to be weak because conscious processes would work in their full performance.
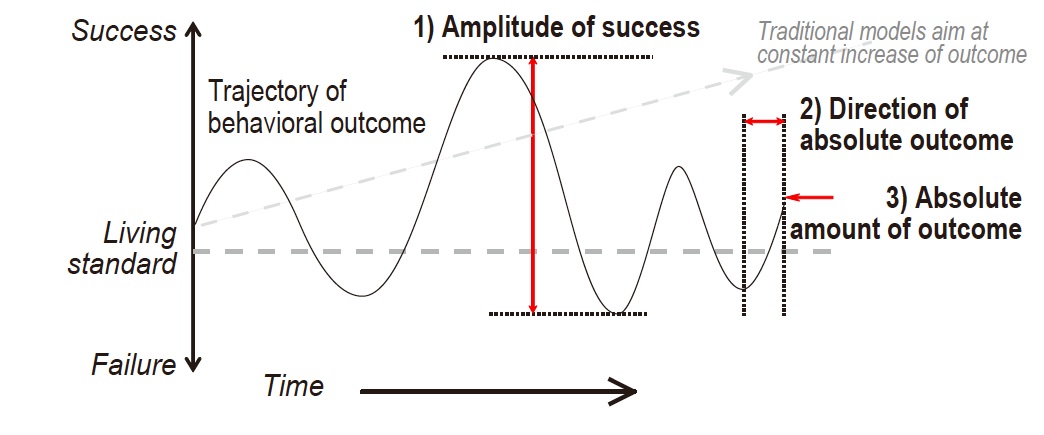
Working of MSA-based intelligent autonomous agents:
The main functions of an MSA-based intelligent autonomous agent that aids the achievement of a happiness goal would include:
- Promoting a state in the autonomous-automatic behavior control layer to a state in the conscious layer,
- Supporting decision making, and
- Activating interaction between the conscious layer and the unconscious autonomous-automatic behavior control layer.
References
Morris, D. (2006). The nature of happiness. 48 Catherine Place, London SW1E 6HL: Little Books Ltd.
Prigogine, I. (1997). The end of certainty. Free Press.